January 18, 2023
Pricing Software Explained
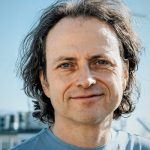
Professionals often think that pricing is just about “do I increase the price by 5%, 7%, 10%?”. They wonder, how hard can that be?
But if you go into the details, the field is, as broad as e.g., the transportation domain. In transportation, you have companies building ball bearings, you have companies operating taxis, and companies building rockets, and it’s all transportation in a sense.
In pricing, you have a wide variety of challenges and solutions that range from gathering pricing data. For example, there are companies that run crawling bots on the internet that collect and compare prices and the availability of products. There is pricing optimization software for companies that have an extensive portfolio of products, and they need to be able to efficiently price all their say “blue products” 5% above their red products. They need to be able to manage and efficiently increase, decrease or differentiate their prices.
There is also automation in pricing. When you’re a company that has a sales force of a couple of hundred or a thousand salespeople, and you want to have consistent pricing across your team, then you need software. That’s obviously massively supported by some software that tells the sales force if your price should be 100 whereas if you have this other type of situation, you should price at 90.
Then there is the field of price optimization lists. It offers transparency and how customers’ demand will react if you change something in price or you remove a product from your portfolio or add a product.
With that transparency, companies can make better pricing decisions because they know that when they add a product, that will increase their revenue by X percent but reduce the profit by Y. In essence, this offers transparency, and that can be used for optimization.
In essence, there are three basic pricing optimization software categories
“Survey-based Price Optimization” is typically not referred to as Pricing optimization software. Platforms like Supra.tools are actually software that conducts standardized unique survey methods and then helps to find the profit-maximizing price optimization. They are software, but the core value comes from the information that is extracted from customers’ survey responses. We exclude this here for clarity.
Let’s dive a bit deeper into the challenges behind the different pricing optimization software features. There is information gathering, and there is price optimization management which means that you need to manage a massive complexity of prices. Then there is the management of the complexity of individual price negotiations and processes. Finally, there is the topic of price optimization and simulation.
What are the different basic needs behind this, and in which industry can we find those needs?
You have a degree of complexity and complexity can come in the form of a large portfolio, it can come-in in the form of many customers, who require differentiated prices. This is what you typically have in a B2B environment, where you have individual negotiations on the price that adds to the complexity, In contrast, a Netflix with 250 million customers comes with two or three price points.
Software tends to gravitate to areas with high complexity. Most suppliers go to areas where you have these individual situations where you need customer-specific price optimization, and you need this many times a day for each salesperson for hundreds or thousands of salespeople and many countries. Those are situations where traditionally, most of that software solutions gravitated to.
A different area is the e-commerce field around Amazon marketplaces, Millions of products change prices daily. These are obviously situations that can’t be managed manually but require some sort of software that steers them. In the most straightforward way, you have something more rule-based where you have, simple rules, which say, “we will always follow our competitors’ price.” With specific software, one can choose “we always stay 2% behind our key competitor in the market.” That is a task that asks for applying software.
Those are the traditional areas of pricing optimization software: high complexity either in the form of many products, or many price changes (e.g. dynamic pricing).
Newer to the space is software that does price modeling: understanding the price demand elasticity based on statistical analysis from sales and other data. Before we dive into this more deeply, let’s look into some applications
What do companies do when they don’t have pricing optimization software, and what did they do before?
Most of them have straightforward pricing rules in place and manage it with Excel and or their ERP. For example, spare parts – companies have hundreds of thousands of spare parts, large automotive companies, for example. They have an initial pricing approach, typically cost-plus pricing, where they know their costs, and then they add some percentage on top. This percentage may depend on the customer value they assign to the product. This is based on expert judgment – not evidence.
Over time they increase the prices by, say 3% – year by year. That produces a number of problems because e.g., the costs change massively or the need for different products changes drastically. If you then compare, different countries that priced independently over a more extended period of time, for many years, you will see eventually that the same product costs twice or three times as much in one country as the next in the case of spare parts where people don’t look too much in the details.
You get these vast differences if you let rules run independently. There’s no reason behind the differences other than that, one of them got increased by 5% each year, the other by 3%. These things produce massive chaos because there are very few forces that work against them. One might be a gray market, if a clever merchant finds it out, he buys it in a cheaper country and exports it to the more expensive one. With an extensive portfolio, it’s tough to figure out where the flaws are.
The different working fields and tasks of pricing optimization software products are as described pricing data gathering, managing complexity, helping in individual negotiations, and price optimization. Which companies should focus on the last part, on pricing optimization using pricing software for optimization?
It’s a newer field. Today there is not much software on proper pricing optimization. Companies that have true optimization in place, tend to have products that are super important, like an iPhone or a new Volkswagen Model where the pricing decisions is just a multimillion-dollar decision, and it makes much sense also to spend large amounts of money on market research and to figure out what’s the best way to price these products is.
Price optimization software becomes apparent when you need to optimize the profitability of many existing products regularly.
We need to be cautious with the term “optimization”. First of all, companies use it even when they just apply fixed pricing rules. Proper pricing optimization needs some kind of modeling. It needs some kind of exercise that aims to understand the willingness to pay and price elasticity.
When we say “price optimization”, it’s not clear what we mean, for another reason. Is it revenue optimization? Is it profit optimization? Or are you optimizing for profitable growth?
When we say “price optimization”, we need to define what you’re actually optimizing. In a straightforward case, where there is just one product and in which you know everything about the product, then it’s easy to see where the revenue optimum is.
It is where price elasticity is -1, and the profit optimum is where price elasticity times margin is “minus 1”. That’s a straightforward rule, but it only applies to one product case and if products are independent.
If you have a portfolio, it’s already different. If you have competition and they might respond to what you do in pricing. The Nash equilibrium case is where you have different parties optimizing the pricing and then the other optimizing again, and so on, and then at some point, you reach an equilibrium where no one can improve further.
That’s what we would call “optimization” dynamically. First, you need to define what to optimize. Then you need to understand the type and dynamics of your market.
Another angle is to also alter your product portfolio structure to optimize for revenue or profits or both: What happens if you change your product, if you add a product, if you remove a product, and then you identify among all of these different cases the case that optimizes your profit? That’s what we would call “optimization” using a market model.
The term price optimization is however used more loosely.
Is there an industry where pricing and profit optimization are less of an issue? Thinking of commodities, retailers or companies sell on Amazon. They may just need to meet the competition.
The sweat spot for price optimization is in cases that are a bit complex and where products are somewhat differentiated so that customers choose between different brands.
There are companies that set a price to a limited customer group, or there are e.g. Telco companies that want to make an upsell offer to existing customers, that currently have a specific product already. This means that the willingness to pay or their preferences are somewhat known as the brand already knows something about the customer because they have already bought a number of products in the past.
In this case, when you are making your customers an offer, people tend to decide on that offer and tend not to look at too many competitive offers in this situation. This is different from the case when you buy something new.
Say, you are at a retailer searching for retail price optimization, in front of the shelf and you choose a bottle of wine where you look at all the different options. Then alternative competitors are much closer than in a situation where you just look at the specific offer you get as an existing customer.
What you will realize in these cases is that these are cases where the standard offer is often very far away from the optimum. The preferences of this particular group who have a particular buying history are very different from the general population and what’s optimal for them can be very different from the overall optimum.
In consumer goods where you may want to sell spaghetti at a retailer, the optimal is not going to be twice the price or half the price of the competition. Still, price optimization is still hugely important as more minor changes have a massive effect on the bottom line and top line.
Most of us know the examples where you have a bottle of water at a retailer, for 50 cents. For that same bottle at the airport, you pay 350 cents, and at the top of Mount Everest, you pay 50 dollars. That’s the context part – in this example the relative price level at the location.
At the airport, the willingness to pay is not really 350. It is just because of the monopoly situation that higher price points are optimal price points. The environment and the selection of products that are being offered in this situation have an impact on what the proper pricing is. If you’re selling a product, the optimum price might be 100. If you have a competitor who would offer something similar, maybe the optimal price goes down to 60.
Let’s look at the methods behind pricing, and profit optimization. Besides econometric modeling or flexible machine learning modeling, there is a new approach around call “virtual customer”.
“Virtual Customers” is a technology that fits well into the time and the methods that are currently available. The basic idea for this is that it looks to understand customer behavior as realistically as possible.
If we have a linear demand function where the maximum sales are 100 units, at 1€ and at 11€ we sell nothing. What that really means is that you lose 10 customers with each Euro you increase.
In the linear case, the distance between these potential buyers is always the same. If you have something like an exponential demand function, it’s pretty different because it gets very narrow at some point where the people are in. But for simplicity, let’s stay with the linear demand function. What this really means is that you have one person for every 10 cents in this example.
The idea with Virtual Customers is actually to rethink what the demand function means. It expands the idea of having one willingness-to-pay for a product at a price towards having an unlimited number of customers who have preferences for different products (not one), different attributes of the product, and so forth. Virtual customers or virtual shoppers have preferences or willingness to pay and specific behaviors.
Some are susceptible to price thresholds, others are not, and they’re all connected by a joint distribution. People who like large cars or who like strong engines might also prefer a BMW over a Volkswagen.
In a sense, they are similar to buyer personas, but buyer personas, you typically have three, four, or five of them. Why? Because as humans, it’s difficult to keep more than three, four, or five concepts in our heads at the same time.
Computers don’t have that problem. Virtual Customer Systems can have 10s of thousands, hundreds of thousands of these virtual shoppers, and the computer can go through each one of them and decide what that virtual shopper would buy in a specific situation.
The idea is that we create a statistical model of the behavior of these virtual shoppers, each one has a selection of preferences for different attributes like brands, and product sizes. Some prefer the middle price option in a good, better, best scenario. Some are indifferent to it. And with all these preferences, they make pricing decisions. We can “show” these virtual shoppers a portfolio. We can change a price. We can add a product, remove a product and simulate how that affects the sales.
The tricky part is getting the suitable parameters into the equation, assuming the right preferences for each of the thousands of virtual shoppers. Typically many different techniques and data are used to derive suitable parameters based on empirical data. We can interpret sales data, as well as survey data such as Conjoint or Implicit Price Intelligence survey outcomes. All of these typical techniques and data measure customer behavior.
The approach builds a model of how a group of customers behaves and this is created using different measurements of customer behavior. What we want to measure is how this group of potential customers will behave in alternative scenarios. We need to understand what their preferences are, and what they would buy. To understand this, we have different methods of measuring customer behavior. For example, looking at their past behavior in a store or asking consumers research questions.
All of these different measurement techniques have advantages and disadvantages.
This illustrates it: If you want to understand the weight of a person, you can put them on a counterweight, or you can drown them in water and see how much water they displace. By knowing the weight of a kilogram, you can compute the weight of the person. In the same sense, as there are different ways to measure the weight of a person, you can measure the preferences of a group of customers in different ways. They all have different advantages and disadvantages. What the virtual shopper approach is doing is integrating all of them and putting them in a coherent model that essentially makes the best use of these different sources.
Pricing modeling is needed for optimization. The conventional approach is finding a formula (a global set of parameters) that can understand the demand based on specific prices and offerings. This formula represents how the market as a whole would react.
The virtual shopper approach is decomposing this into markets of one. The advantage of it is that you can now use multiple data sources to make this more robust.
If you measure the weight of a person in different ways, you would expect them to be roughly the same. The estimation of the willingness to pay is much more complex, and the results are noisy.
With something like “price elasticity” it’s different; you only look at a specific product. If you have, say the price elasticity of a glass of water is -2, you look at that glass of water. But in reality, it depends on the price of other drinks that are available. If the context shifts, price elasticity shifts.
If you decrease the price of other drinks, they draw demand from our glass of water, and that shifts its demand curve. That’s a challenge, especially for products that are primarily determined by the competitive or context setting, which might be subject to change.
The virtual shopper approach allows us to be more realistic. As virtual shoppers can react to any kind of change in an offer. If a competitor increases prices or if a competitor removes a product.
Like with every model, it all depends on the quality of the input data. If the inputs are not precise, it reduces the validity of the model. Assume customers come from two different channels, but one channel was missed in aggregating the data. If the past data isn’t correct, then this is difficult.
Therefore, the fundamental data source preferred is historical sales data. There are some things that are difficult to figure out with historical data too.
For example, if you have a particular brand, and it only offers its product in a specific size. Let’s say you sell orange juice, everyone else sells it in half-a-liter and one-liter bottles, and there’s one brand that only sells 0.7 liters, and they are the only ones. Then with historical data it is, to some extent, difficult to say, is it the 0.7 attracts people or is it that brand? That’s something that can be better found out with market research techniques.
For so many industries, let’s say Telco, for example, you have something like technical inflation, that the willingness to pay for like a gigabyte of data declines over time or it’s because more becomes available.
Other challenges with historical data arise when one-time events influence data. In the COVID case where preferences changed or with the energy increasing energy costs in Europe, you have effects that are a bit more discontinuous when you look at the past.
There are quite a few more situations where that past data is not enough. This is first the launch of new products and the introduction of new packaging designs or sizes. Also if brands want to reduce or increase the price in a way that had never happened.
Interpolation is less of a problem. In the orange juice example, let’s say, historically, we’ve had 0.6 Liter and 0.8 Liter, then to interpolate to 0.7 is pretty straightforward. That would reasonably be allowed.
Extrapolation can become a problem. If we have everything from 0.5 to 1 liter as data and want to simulate 1.1 or 1.2 Liter, this will be predictable and accurate. But if you go to 2 liters, that’s much more difficult. What is also tricky, is if you have a new category. new packaging, that hasn’t existed before. Also, if you introduce a new brand is something where it makes much sense to leverage market research tools.
Today pioneers use the latest pricing survey tools that use Neuroscience + AI
Interested professionals can use some tools even for free and explore on its own
You can access supra tools free here.
Which price optimization software exists? Who should watch out for which type of software? Who should reach out to alternative approaches?
There’s a vast variety of pricing optimization software. Pricing is probably as broad as the “transportation” category. There is a massive number of different software that are available focusing on different areas: management, automation, collection of insights and competitor prices, and modeling-based optimization.
The most difficult and also the most exciting area is optimization. Before you can perform optimization, it is helpful to understand how the market reacts to changes and how demand reacts to price or offer changes. But first of all, you need to answer the question of what optimization actually means. Are you optimizing revenue, profit, or market share?
In many circumstances, it’s not enough to just let pricing optimization software work with the data you have or are crawling from the web. Instead, then you need market research tools. With Implicit Pricing intelligence, there is now a technology available that makes this research highly accurate, easy to set up, inexpensive, and fast to execute.
When it comes to pricing new products or packaging, extrapolated price levels, or you simply do not have access to reliable first-hand data, then pricing optimization software should be complemented by market research and particularly by Implicit Pricing Intelligence solutions.
In my email newsletter “Pricing Insights” I cover the whole range of pricing insights solutions-from Garbor Granger to Conjoint, from NeuroPricing to Pricing Software Systems. I describe the application in various fields from new product pricing to promotion, from brand premium to feature pricing.
Keep up to date here.